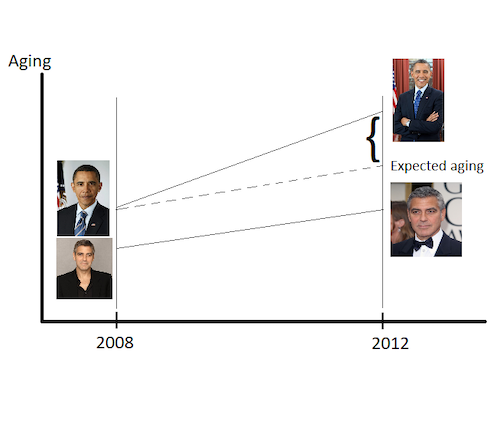
Understanding Matching and Diff-and-Diff
by Lily An and Kristen HunterDoes becoming President of the United States cause you to age faster than you would have otherwise, if you had not become President?
Media sources have claimed that presidents age more quickly while in office. The British tabloid The Daily Mail asserted “American presidents age TWICE as fast while in office” (Daily Mail Reporter 2011), and Business Insider commented on the “striking difference” between President Barack Obama’s 2008 presidential portrait following his election and his 2012 presidential portrait before his second term (LoGiurato 2013).
We can consider the question of presidential aging ourselves by examining the aforementioned presidential portraits of Obama.
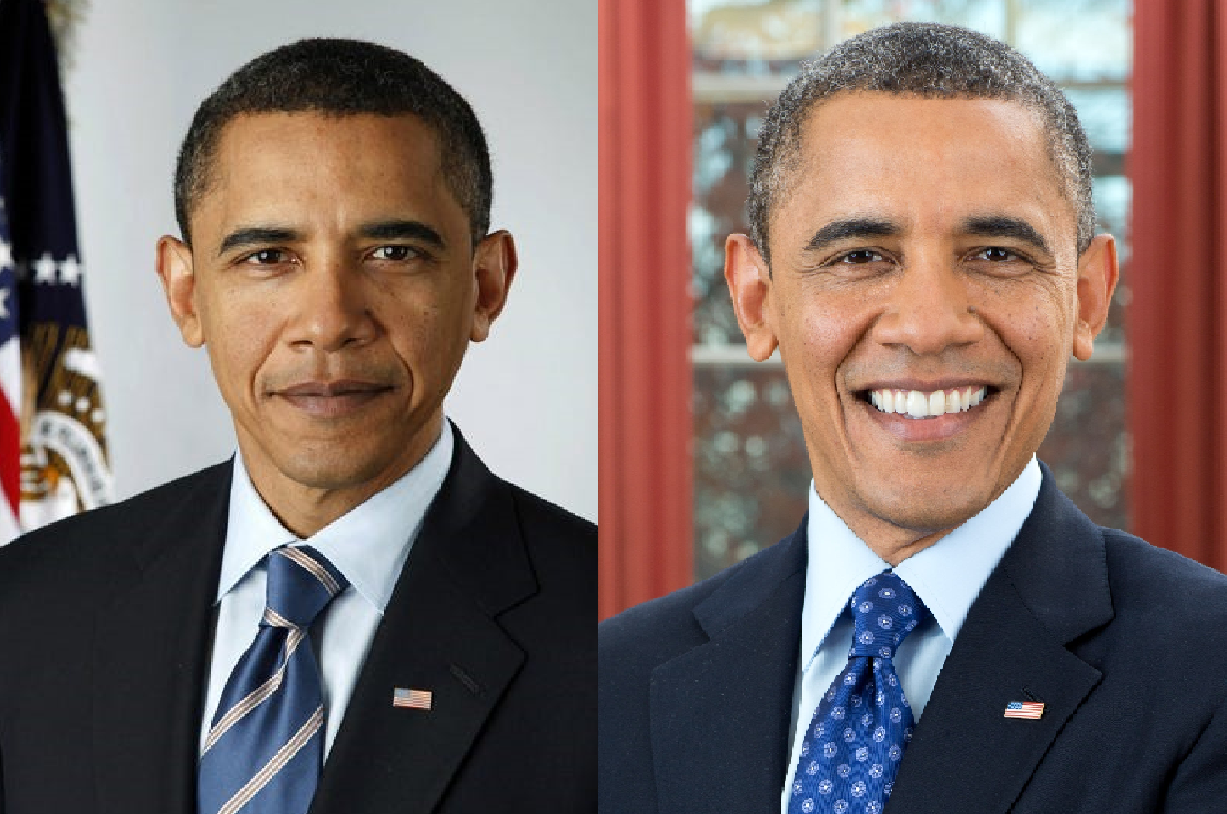
Looking at the differences in hair color and under-eye bags across portraits, it does seem that President Obama aged substantially during his first term. Does this mean becoming President accelerates aging? Did Obama indeed, as the Daily Mail claims, age twice as fast as he otherwise would have?
Comparing these two pictures alone does not allow us to answer our question. We know that President Obama aged at least a little over his first presidential term because, after all, time passed. But was his aging process the same as it would have been if he had not become president? In order to figure out whether Obama’s presidency aged him faster than a different job, we need to compare him to other people. Causal inference is fundamentally a study of comparisons: through comparisons we can try to estimate the effect of becoming president on Obama’s aging.
Diff-in-Diff
We never will know what would have happened to President Obama’s aging process had he lost the presidency in 2008. However, what we can do is compare pre- and post-presidency pictures of him to pictures of people who were not president over the same time period. Our outcome is the amount the subjects of the pictures have aged: have they gone grey? Gained wrinkles? Lost hair?
By comparing the aging process in different people, we are intuitively using a method called Difference-in-differences (DID) to estimate the aging effect of becoming president. Diff-in-diff, as it’s colloquially known, attempts to break down overall aging into two parts: Obama’s aging that would have happened naturally over those four years, and the ‘extra’ aging that is specifically due to becoming President.
For our example, the DID method compares the speed of aging of a president to the speed of aging of someone who was not a president. We illustrate the main concept of DID in the figure below. Let’s compare Obama to someone who was also age 47 in 2008: the actor and husband of Amal Clooney, George.
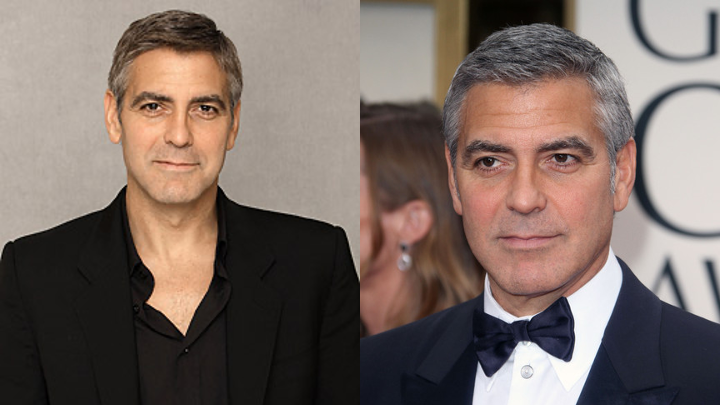
Although Clooney’s hair also turned white over 2008-2012, his smoldering good looks remained relatively youthful. Obama, by contrast, arguably seems to have aged more than Clooney. Let’s also acknowledge that at the outset of our date range in 2008, Obama and Clooney looked slightly different with regards to age. To account for this, we’ll place both men at different starting levels of aging in 2008.
To compare the difference between the two men, first we track how much aging we see in Clooney’s pictures from 2008-2012.
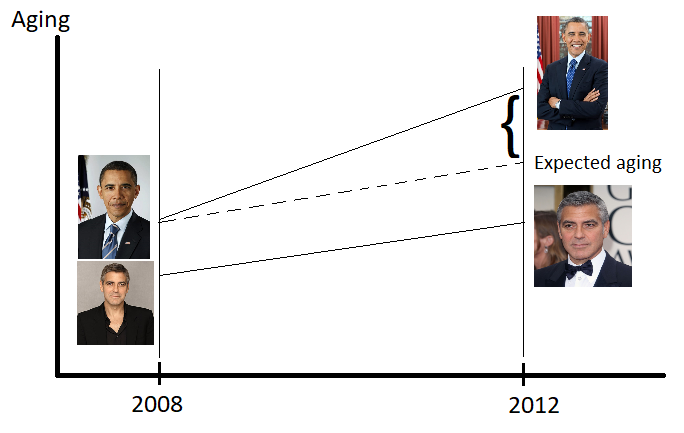
From this trend, i.e. the lower solid line, we can assess the ‘non-president’ amount of aging for Clooney from 2008-2012. Second, we plot how much aging we see for Obama: this is the upper solid line. Finally, we use the aging trend from the non-president to predict where in the aging process President Obama would have been if he had not been president, which is the dotted line. By taking the difference between the upper solid line and the dotted line in 2012, we can see to what extent Obama aged above and beyond the non-president amount. This bracketed gap is our estimate of the causal effect of becoming president on aging.
Taking a step back from this picture, we could be a bit more thoughtful about who is in our non-presidents group. Importantly, we need to ask: does Clooney make sense as our comparison to Obama? Though they are similar in age, Clooney has a fundamentally different life than President Obama. We don’t know how wrinkles are affected by having copious amounts of money and other benefits of being a successful actor.
DID relies on an assumption commonly called ‘parallel trends,’ which is that if none of our subjects had become president, then they would all have experienced the same aging trends. Although we do not have to assume that Obama and Clooney start out at the same point, we need to assume that Obama would have aged the same amount as Clooney if he had not become president.
We may be more likely to meet this assumption if we compare a pair of people who are extremely similar, with their only difference being the minor life change of becoming President. Because we do not actually believe that Clooney and Obama are fundamentally similar people given that actors may not visibly age at the same rate as politicians, we would not want to have George Clooney as our ambassador of all non-presidents. Thus, our use of diff-in-diff may be a little unsatisfying here. This example highlights that we need to be careful about selecting our comparison group (in this case a comparison person) before estimating the causal effect of becoming President by using diff-in-diff.
Matching
The strategy of finding people as a point of comparison is called matching. The goal of matching is to find people who are similar on background characteristics, but who differ only in terms of our factor of interest (here, becoming President). Creating matches helps us to estimate a more valid and robust causal effect. In our context, we are stepping back to find a good match for Obama, after which we would use diff-in-diff to compare aging outcomes across the pair. If President Obama’s aging looked the same as a comparable person who had not become president, then becoming president most likely did not accelerate his aging. On the other hand, if President Obama’s aging looked more intense than a comparable person who had not become president, then it is more likely that becoming president did accelerate his aging.
One potential comparison is Senator John McCain, who lost the presidential election to Obama in 2008.
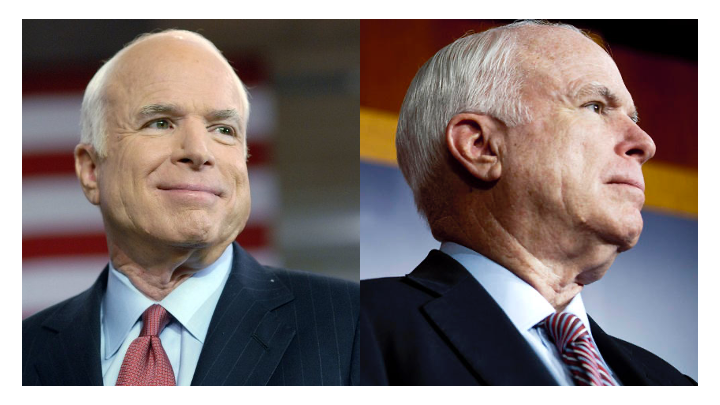
He may be a good potential comparison because they have similar career trajectories: they were both senators who became the leading presidential candidates. When looking at these pictures of Senator McCain to assess the amount he aged, he seems to have fared better than Obama in the intervening four years: he aged, but perhaps not as much as President Obama. Any wrinkles in 2012 were also present in 2008.
However, maybe the difference in aging between President Obama and Senator McCain over this time period is not due to the presidency at all, but merely due to their different starting ages in 2008 (47 for Obama and 72 for McCain). We can imagine that people age at different rates depending on their stage in life, so to better control for this, we can go farther back in Senator McCain’s past. Let’s observe Senator McCain’s aging process when he better matches President Obama’s ages in the presidential portraits: from the age of 47 in 1983, to 52 in 1988.
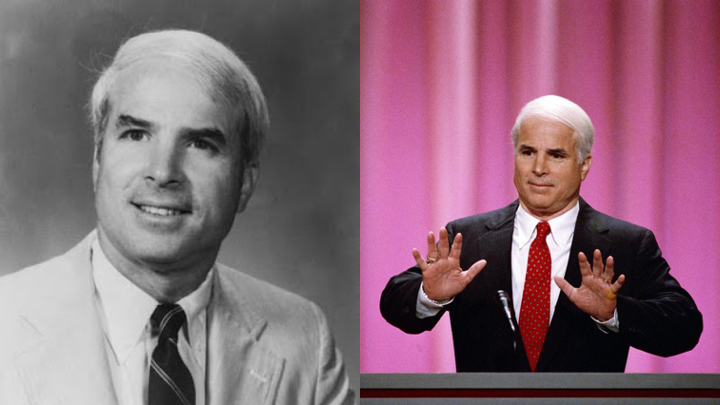
McCain’s appearance over these ages seems to have changed to a smaller degree than Obama’s, implying that the presidency did accelerate President Obama’s aging.
Although we have now controlled for starting age, a 1983 Senator McCain may still not be satisfying to you as a match to 2008 Obama. If we were to move forward with this match, we would be assuming that people aged at the same rate in the 1980s and in the 2000s. But, the 1980s were a much different decade–perhaps 47 year old McCain was practicing aerobics and not ingesting microplastics, allowing him to keep his face free of the wrinkles that Obama accumulated between 2008-2012. Most likely we would want to find a match from roughly the same time period as Obama’s portraits to get rid of this ‘decade effect.’
These examples of McCain and Clooney have very quickly shown us one of the main challenges of matching. An ideal match is similar to our person of interest in every way that matters with respect to aging, except that they differ on the factor of interest (i.e. being president). Deciding what matters for the match is tricky, though, and if the matches differ too much, we will have little faith that any difference in outcomes is due to the factor of interest–instead it could simply be due to the different characteristics of the people in the matched pair. Regarding our examples, we would probably reject our current proposed matches as not being very good comparisons for Obama. John McCain differs too much in age/decade, and George Clooney differs too much in occupation and lifestyle as an actor.
Conclusion
To examine the issue of rapidly-aging presidents, we have explored two methods in the causal inference toolbox: matching and diff-in-diff. Matching is the process of constructing a valid comparison group which allows us to better estimate a causal effect of interest. DID is a method of estimating a causal effect by comparing the change between two groups over time. We likely would need both of these methods to approach a satisfying result to our question.
Although we have examined these methods in the context of presidential aging, there are a variety of problems with our current strategy that affect our ability to come to a causal conclusion. First, let’s consider one problem we can’t really fix: we have asked a question about American presidents, which fundamentally restricts our sample to a pool which is not only small, but even worse, collected over many decades. With such a small and motley crew, it is unlikely we would be able to obtain reliable and strong results. For one thing, it is likely that the causal effect of the presidency on aging varies widely within this group! It is easy to imagine that Grover Cleveland faced a much different aging trajectory from Barack Obama. We can’t fix this problem without changing history and the U.S. Constitution to add more presidents to our data.
A second challenge is that we would need to find a high-quality match, or potentially multiple matches, for each U.S. president. This challenge is one that we could potentially overcome, but that is not to say it wouldn’t be difficult. So far, we have not even found a satisfying match for Obama! For each president, we would need to find a person (or people) from the same era who is (are) fundamentally similar in ways that are important to aging, but just so happened to not become president.
A third problem is that our current method of measuring the outcome involves us squinting at pictures and coming to some subjective judgement of aging, which isn’t what we would want to do in a real study. Rather, we would want to have a more rigorous and clear definition of aging, whether determined algorithmically or still by hand, but at least with multiple people judging.
To summarize, in a perfect setting, we would have (1) an infinite number of presidents, (2) a set of non-president candidates who are perfect matches, and (3) a valid and reliable measurement of aging. We never have such ideal conditions, but a good study would at least involve a large sample size, well-constructed matches, and some careful thought about how to best measure the outcome of interest.
So, did Obama becoming president age him above and beyond typical aging? For now, it appears we are unable to very seriously determine the answer to our question. Thanks, Obama (Know Your Meme 2013).
Appendix: Further exploration
DID is one amongst many methods of causal identification that researchers use to estimate a causal effect. Specifically, DID is used when we observe trends over time. If instead we were to ask whether being president causes people to kiteboard with billionaire Richard Branson (Bowerman 2017), we would not want to use diff-in-diff, but instead a method that does not require panel data.
One fundamental assumption of DID which we have discussed is the parallel trends assumption. In our example, we used the parallel trends assumption to project that Obama’s aging trajectory would have been the same as George Clooney’s if Obama had not become president. Sometimes researchers try to conduct tests to evaluate the validity of the parallel trends assumption, but we must tread carefully when doing so; see Bilinski and Hatfield (2019) for a discussion.
Matching can potentially help us make the parallel trends assumption more plausible, as we are much more likely to believe that similar people would follow similar trends! One reason we rejected Clooney as a good match is that we were skeptical that his trend would be the same as Obama’s non-president trend. However, we also have to be careful in using matching in combination with DID. Daw and Hatfield (2018) point out that in certain cases, matching before DID can actually induce bias in situations that would have been unbiased otherwise.
For an introduction to DID, see Angrist and Pischke (2009); for a briefer, more technical introduction see Imbens and Woolridge (2008); for canonical examples, see Ashenfelter and Card (1985) and Card and Krueger (1994). For an example of more recent work that generalized DID into a broader framework, see Doudchenko and Imbens (2016).
Though we examined matching solely in conjunction with DID, it is a widely-applicable strategy which can be used to answer causal questions. Matching is a framework for creating treated and control groups which are balanced in their background covariates. Creating balanced groups can make the estimation of the treatment effect less biased. After matching, the analyst can use whatever estimation strategy makes sense for the problem, from taking a simple difference in means between the treated and control groups, to fitting a linear regression, to using a complex Bayesian model. There is a rich research literature surrounding matching; for a clear introduction and overview of matching methods, see Stuart (2010).